How is autonomous driving technology being evaluated? The development and testing of vehicles capable of operating without human intervention are critical to the future of transportation.
Autonomous vehicle trials are a vital stage in the process of introducing fully automated driving systems. These trials involve controlled, real-world testing, where vehicles navigate varying road conditions and traffic situations under a variety of environmental circumstances. They are designed to evaluate the safety, reliability, and robustness of the technology. Examples include navigating complex intersections, adjusting to unexpected situations like sudden stops or lane changes, and managing varying weather conditions like rain or snow. Detailed data analysis of these trials helps refine algorithms and system protocols.
These trials are crucial for advancing the technology. Success in trials often paves the way for wider adoption, potentially revolutionizing transportation by enhancing safety, reducing congestion, and making mobility more accessible. The data gathered informs further technological development, leading to a potentially safer and more efficient driving experience. Trials also identify shortcomings in the algorithms and hardware and suggest improvements to the underlying systems. Furthermore, they provide valuable feedback to engineers on how to enhance the performance and reliability of the systems in different environmental and road conditions.
Transitioning to the main article topics...
Full Self-Driving Trial
Evaluating the efficacy and safety of fully automated vehicles requires rigorous testing. Trials provide critical data for system improvement and public acceptance.
- Technological advancement
- Data acquisition
- Safety validation
- Environmental adaptation
- Regulatory compliance
- Public perception
- Ethical considerations
These key aspects are interconnected. Technological advancement fuels data acquisition, which informs safety validation and adaptation to varying environments. Successful trials demonstrate regulatory compliance, shape public perception, and raise ethical considerations about automated systems' use. For instance, a trial might discover a software glitch impacting braking in adverse weather, highlighting the need for further adaptation. Successful trials demonstrate the system's ability to safely navigate complex situations, influencing public perception and fostering acceptance. Ultimately, ethical frameworks must accompany technological progress in autonomous driving to ensure responsible implementation.
1. Technological Advancement
Technological advancements underpin the feasibility and safety of full self-driving trials. Progress in sensor technology, artificial intelligence (AI), and vehicle control systems directly affects the capabilities and reliability of autonomous vehicle systems undergoing testing. These advancements determine the types of scenarios the vehicles can handle, influencing the scope and design of trials.
- Sensor Technology Advancements
Improvements in sensor accuracy and reliability are critical. Sophisticated cameras, radar systems, and lidar provide more detailed and comprehensive data about the environment, enabling more precise object recognition and decision-making. For instance, enhanced lidar systems enable vehicles to perceive objects more accurately in low-light conditions or heavy fog, extending the scope of testing environments. This increased accuracy directly impacts the vehicles' ability to navigate complex scenarios and react appropriately in challenging situations.
- AI Algorithm Refinement
AI algorithms are central to autonomous decision-making. Continued development of more sophisticated algorithms allows vehicles to learn and adapt to unpredictable situations and varying traffic patterns. This learning process, often based on vast amounts of data from trials and simulations, enables vehicles to improve their response times, anticipate potential hazards, and handle unforeseen events with greater efficacy. Algorithms capable of recognizing nuanced pedestrian behavior are crucial for safe interactions in complex environments.
- Vehicle Control Systems Enhancement
Advanced control systems are vital for enabling safe and reliable maneuvers in real-world conditions. Improvements in braking, steering, and acceleration systems ensure precision and responsiveness in critical situations. This enhances the vehicle's ability to maintain stability and execute intricate maneuvers with greater consistency, enabling more rigorous trials that push the limits of autonomous driving capabilities.
- Data Management and Processing
Effectively processing and managing vast quantities of data collected during trials is essential for refining autonomous vehicle algorithms. Improvements in data analysis methodologies allow for faster identification of patterns, anomalies, and areas requiring further development. This capacity is crucial for improving the vehicle's decision-making process and for identifying system weaknesses in real-time, enabling quicker responses to challenges encountered during testing.
In summary, each facet of technological advancement directly influences the success and sophistication of full self-driving trials. The interconnected nature of these improvements is vital to pushing the boundaries of autonomous driving, culminating in systems capable of safely navigating a wider range of environments and situations.
2. Data Acquisition
Data acquisition forms the bedrock of effective full self-driving trials. The quality and quantity of data gathered directly influence the algorithms' accuracy and the system's ability to adapt to real-world conditions. This data underpins advancements in autonomous vehicle capabilities.
- Environmental Data Capture
Trials require comprehensive data encompassing diverse environmental conditions. This includes variations in weather (sun, rain, snow, fog), light levels, road surfaces (wet, dry, icy), and traffic density. Data from these varied conditions are essential for calibrating the system's response to fluctuating environmental factors. Examples include detailed sensor readings during various weather events to train the algorithms to handle adverse conditions. Inaccurate or incomplete environmental data can lead to system malfunctions in real-world driving scenarios.
- Traffic Pattern Analysis
Detailed data on traffic patterns, including speed, distance, and maneuvers of other vehicles, is critical. This encompasses diverse traffic situations, such as merging lanes, intersections, and highway driving. This dataset helps the system learn how to anticipate and react safely to various traffic behaviors, making navigation more reliable. Examples include analyzing thousands of hours of real-world driving data to identify consistent traffic behaviors and potential hazards, fine-tuning predictive models.
- Object Recognition Refinement
Data on objects within the environment are crucial for the system's understanding of the surroundings. This includes data on vehicles, pedestrians, cyclists, and various stationary objects. Analyzing large datasets of object recognition ensures the system identifies these entities accurately and precisely, particularly in challenging situations like heavy traffic or obstructed views. Examples include images and sensor readings used to train the system to differentiate different vehicle types or identify pedestrians crossing the street at varying speeds and angles. Inaccurate object recognition poses a significant safety risk.
- System Response Calibration
Data detailing the system's response to diverse stimuli are necessary for assessing its performance and efficacy. This includes data on acceleration, braking, steering, and overall response times in a variety of scenarios. Examples include collecting data on the system's reaction to unexpected obstacles, sudden lane changes, or emergency braking situations to ensure safe and efficient response mechanisms. Incomplete or inconsistent system response data may lead to unpredictable or dangerous outcomes in actual driving environments.
In conclusion, data acquisition is an indispensable component of full self-driving trials. By meticulously gathering, analyzing, and refining data from diverse environmental scenarios, traffic patterns, object recognition, and system responses, trials ensure the system adapts effectively to real-world conditions. This comprehensive dataset is vital for developing a robust and safe autonomous driving system.
3. Safety Validation
Safety validation is paramount in full self-driving trials. Rigorous evaluation of the autonomous system's performance, robustness, and reliability under various conditions is indispensable. Without thorough validation, deployment of such technology in real-world settings is unacceptable and potentially dangerous. Trials serve as a crucial testing ground for identifying and addressing potential vulnerabilities within the system, ultimately contributing to the safe operation of autonomous vehicles.
- Scenario-Based Testing
Comprehensive trials simulate a wide spectrum of driving scenarios. This includes diverse road conditions, varying traffic densities, complex intersections, and unexpected events like sudden stops or pedestrians entering the road. Data gathered from these simulations assists in identifying areas where the system falters or performs inadequately. For example, testing in adverse weather conditions allows assessment of the system's response to reduced visibility and slippery surfaces. Correctly identifying and addressing these vulnerabilities are crucial for the safety of occupants and other road users.
- Redundancy and Fail-Safes
Trials assess the system's ability to maintain functionality even when encountering critical failures in components or algorithms. The presence of redundant systems or fail-safe mechanisms is crucial. For example, verifying that the system can revert to a predetermined control mode when a critical sensor malfunctions. This ensures continued safety and prevention of hazardous incidents in real-world operations.
- Human Intervention Protocols
Trials meticulously evaluate the effectiveness of human intervention protocols. The system's ability to seamlessly hand over control to human operators in critical situations needs to be fully validated. For instance, observing how the vehicle reacts to the activation of the manual override mechanism. This includes examining response times during unexpected situations and ensuring the system's control relinquishment procedures are instantaneous and effective.
- Post-Trial Data Analysis
Detailed data analysis after each trial is crucial. Statistical evaluation of performance metrics, such as accident rates, response times, and control transitions, identifies system vulnerabilities or opportunities for improvement. For example, analyzing collected sensor data from various scenarios helps discern patterns related to specific error triggers. This data-driven approach leads to targeted development and correction of safety-critical components.
These facets of safety validation are interwoven. Rigorous scenario-based testing, complemented by redundancy checks and human intervention protocols, ultimately leads to improved reliability. The system's safety is not just validated through the trials themselves, but critically by the thorough analysis of the collected data. Continuous refinement and improvement, driven by safety validation insights, are essential for the responsible development and deployment of fully autonomous vehicles.
4. Environmental Adaptation
Environmental adaptation is a critical aspect of full self-driving trials. Autonomous vehicles must operate reliably and safely across diverse and dynamic environments. This includes navigating various weather conditions, terrain types, and traffic patterns. Successful trials demonstrate the system's capacity to adapt to these changing conditions.
- Weather Variations
Autonomous vehicles must effectively handle diverse weather conditions, including rain, snow, fog, and extreme temperatures. Trials assess the system's ability to maintain its navigation and safety protocols under these circumstances. Robust sensor systems, capable of accurate object recognition in adverse conditions, are essential. For instance, trials in inclement weather allow evaluation of how well the system perceives objects amidst reduced visibility, ensuring safety and reliable functioning.
- Road Conditions and Terrain
Road surfaces, including ice, gravel, and uneven pavement, influence the vehicle's performance. Trials should encompass various road types to evaluate the system's capability in handling uneven or complex terrains. The system's responsiveness and control mechanisms are tested on different road conditions to maintain stable trajectory, predict and react appropriately to changes in the road's surface. For instance, trials on winding roads or those with sharp curves validate the system's ability to navigate and maintain stability.
- Traffic Dynamics
Autonomous vehicles must adapt to various traffic patterns, including heavy congestion, merging lanes, and unpredictable driver behavior. Trials should involve a broad spectrum of traffic situations to test the system's capacity to predict and respond safely to unpredictable maneuvers by other drivers. Data from these trials helps to refine the system's decision-making capabilities in diverse traffic environments. For example, trials in high-traffic areas provide valuable data for evaluating the algorithms' ability to anticipate and react safely to other vehicles' movements, reducing the risk of accidents.
- Lighting Conditions
Variations in lighting conditions, from bright sunlight to complete darkness, impact the performance of sensors. Trials in diverse light environments are crucial to ensure the system can identify and react appropriately to objects under varying light conditions. This involves evaluating the effectiveness of sensor calibration across different lighting conditions. For example, trials at night or in low-light conditions assess the system's ability to navigate and identify objects safely, enhancing visibility and minimizing risk.
Successful environmental adaptation in full self-driving trials is not just about individual components but about the interconnectedness of these facets. The system must react effectively in any combination of these conditions, as real-world scenarios often present a confluence of challenges. Trials serve to isolate and measure performance in specific contexts, enabling developers to enhance the system's capabilities for handling diverse and unpredictable environmental conditions, making autonomous driving more reliable and safe.
5. Regulatory Compliance
Regulatory compliance plays a pivotal role in full self-driving trials. The development and testing of autonomous vehicles necessitate adherence to established legal frameworks. These frameworks ensure safety, public trust, and responsible implementation of the technology. Compliance standards dictate the types of tests conducted, the data collected, and the limitations placed on the vehicles' operational capabilities during trials. Without a clear regulatory framework, these trials lack legitimacy and public acceptance.
Compliance requirements often stipulate specific testing protocols. These protocols detail the scenarios vehicles must navigate, the environmental conditions under which testing occurs, and the data collected during trials. Adherence to these standards ensures that the data generated is reliable and comparable across various trials. For example, regulations might mandate that autonomous vehicles undergo testing in specific weather conditions or at certain traffic densities. Furthermore, regulations often address data privacy and security aspects related to the enormous amount of data generated during these trials. Clear regulations governing data ownership and usage are critical to ensure responsible data handling, thereby fostering public confidence.Failure to meet regulatory requirements can lead to significant consequences. Trials might be halted, data deemed inadmissible, or even the project itself discontinued. The practical implications of such setbacks are substantial, delaying the wider adoption of autonomous vehicles, potentially deterring further investment in the technology, and impacting public perception. Examples of jurisdictions implementing regulations specifically for autonomous vehicles highlight the growing emphasis on ensuring that testing and eventual deployment of such vehicles adhere to safety and ethical guidelines. This adherence is vital to build public trust and support for the technology. The development and implementation of clear regulatory standards are vital to ensuring safe, reliable, and ethical operations of autonomous vehicles.
In summary, regulatory compliance is integral to full self-driving trials. By establishing standards, overseeing testing procedures, and ensuring data integrity, regulatory frameworks contribute significantly to the safety and ethical development of this technology. Failure to adhere to these frameworks can have significant negative consequences, hampering progress and deterring public acceptance. Consequently, establishing clear, comprehensive, and evolving regulations is critical for promoting responsible innovation in the realm of autonomous vehicles and maintaining public trust throughout the development process.
6. Public Perception
Public perception is a critical component of successful full self-driving trials. Positive public sentiment fosters trust and acceptance, which are essential for the widespread adoption and integration of this technology. Conversely, negative or uncertain public perception can hinder progress, potentially creating resistance to implementation and slowing down advancements. The public's understanding and acceptance of autonomous vehicles directly influences the success of trials, shaping regulations, influencing investment, and determining the technology's long-term viability. Trials themselves act as a key element in shaping this perception, offering the public opportunities to observe the technology in action and form their own judgments.
Real-world examples illustrate the significance of public perception. Early autonomous vehicle trials often faced skepticism and apprehension. Initial concerns about safety, reliability, and the potential displacement of jobs fueled negative media narratives. This, in turn, created a hesitant public. However, as trials demonstrate the technology's progress, improving safety and reducing incidents, public opinion shifts positively. Successful trials that highlight the technology's benefits and minimize perceived risks are instrumental in building confidence and mitigating potential resistance. Furthermore, transparent communication about the limitations and challenges of the technology is crucial to manage public expectations and build trust. For example, if trials reveal specific vulnerabilities, proactively addressing these issues through subsequent trials can reinforce public trust and understanding.
Understanding the connection between public perception and full self-driving trials is crucial for stakeholders. This understanding allows for more effective communication strategies, better-designed trials, and ultimately, a smoother transition toward widespread implementation. Thorough public engagement, clear communication about safety measures, and proactive transparency can directly impact public opinion. By proactively addressing concerns and demonstrating progress through successful trials, the technology can gain public acceptance and support, paving the way for a future where autonomous vehicles become a common part of everyday life. Without considering this dynamic interplay between technology development and public perception, progress will be hampered, and potential benefits will not be realized.
7. Ethical Considerations
Ethical considerations are intrinsically linked to full self-driving trials. The development and deployment of autonomous vehicles raise complex moral dilemmas that demand careful consideration. These dilemmas arise from the technology's potential to impact human life and the intricate choices autonomous systems must make in the absence of direct human control. Defining ethical protocols within the testing process is critical to ensure responsible innovation. The concept of "who is responsible?" in the event of an accident the manufacturer, the software engineer, or the system itself necessitates clear frameworks for accountability and liability.
Real-world examples illustrate the ethical complexities. Imagine a trial where an autonomous vehicle must choose between colliding with a pedestrian or swerving into another vehicle. The algorithm's programming must predefine the priorities in these scenarios, but different approaches may favor different values, leading to controversy and potential legal challenges. How do ethical frameworks account for the varied cultural values and societal norms in different regions when developing these safety protocols? The potential for bias within the algorithms, trained on data that may reflect existing societal biases, underscores the importance of diverse datasets and careful ethical evaluation in the development and testing phases. This underscores the need for ongoing discussions and collaboration between technologists, ethicists, policymakers, and the public. Such collaboration is crucial for establishing universally accepted guidelines for autonomous vehicles.
Understanding the ethical implications is paramount for successful trials. The potential for unintended consequences in autonomous vehicle technology underscores the importance of embedding ethical considerations from the outset of trials. Failure to address these issues may result in public mistrust, legal challenges, and a slower adoption rate for the technology. A robust and transparent ethical framework is thus not just a component of trials but a vital prerequisite for their long-term success. Developing such a framework demands careful consideration of various ethical dilemmas, proactive public engagement, and continuous refinement of ethical guidelines. Only then can the potential benefits of this technology be realized responsibly.
Frequently Asked Questions about Full Self-Driving Trials
This section addresses common inquiries regarding full self-driving trials, offering clarity and context on the testing process.
Question 1: What is the purpose of full self-driving trials?
Full self-driving trials are designed to evaluate the performance and safety of vehicles capable of operating without human intervention. Trials assess the system's ability to handle various environmental conditions, traffic scenarios, and unexpected events. Data gathered from these trials informs algorithm refinement, system improvements, and ultimately, the safe deployment of autonomous driving technology.
Question 2: How are these trials conducted?
Trials involve controlled environments, incorporating diverse road conditions, traffic patterns, weather conditions, and challenging scenarios. Data acquisition, from sensor inputs to operational logs, provides crucial feedback for system enhancements. Rigorous data analysis and simulations are integral aspects of the testing process.
Question 3: What are the key safety considerations in these trials?
Safety is paramount. Trials include redundant systems and fail-safe mechanisms. Protocols for human intervention in critical situations are meticulously tested and evaluated. Data analysis plays a crucial role in identifying and addressing potential system vulnerabilities and in ensuring the technology's reliability and safety.
Question 4: What role do regulations play in full self-driving trials?
Regulations establish standards for testing procedures, data collection, and safety protocols. Adherence to these standards ensures the trials are conducted responsibly, that the data is reliable, and that potential impacts on the public are addressed appropriately. Compliance with regulations is essential for the credibility and future deployment of the technology.
Question 5: How do full self-driving trials contribute to public acceptance of autonomous vehicles?
Successful trials build public trust by demonstrating the technology's safety and reliability. Transparent reporting on trial results, challenges faced, and solutions implemented helps to dispel misconceptions and fosters a more informed public perspective. Positive public perception is crucial for eventual acceptance and integration of autonomous vehicles into society.
In conclusion, full self-driving trials are a crucial step in the advancement of autonomous vehicle technology. They ensure safety, reliability, and responsible deployment while fostering public understanding and acceptance of this emerging technology.
Transitioning to the next article section...
Conclusion
Full self-driving trials represent a crucial phase in the development of autonomous vehicles. These trials are more than mere demonstrations; they serve as a complex and multifaceted evaluation process. The trials meticulously assess the technology's ability to navigate diverse environmental conditions, respond to unpredictable situations, and operate safely in various traffic scenarios. Key aspects, including technological advancements, data acquisition, safety validation, and regulatory compliance, are interwoven and interdependent. The meticulous analysis of gathered data informs subsequent algorithm improvements, ultimately shaping the future of autonomous vehicle technology. Furthermore, public perception and ethical considerations play pivotal roles in determining the long-term success and societal acceptance of fully autonomous systems. Trials serve as a critical juncture, shaping public understanding and expectations, and guiding future development toward responsible integration into society.
The ongoing evolution of full self-driving trials signifies a critical juncture in the evolution of transportation. Successful completion of these trials is essential for ensuring the safe and efficient integration of autonomous vehicles into public roadways. Continued rigorous testing and meticulous evaluation of these systems remain essential for the long-term viability of this transformative technology. Public trust, informed by transparent and responsible reporting, is paramount to fostering widespread adoption. The ultimate goal is not merely technological advancement but the responsible application of this technology to improve the lives and well-being of individuals and society as a whole. Therefore, the journey of full self-driving trials, characterized by diligent testing, ethical considerations, and regulatory compliance, is an ongoing and indispensable part of shaping a future powered by autonomous vehicles.
Buy Holocoin: Top Exchanges & Where To Find It
Vanguard Total Stock Market Index Fund ETF (VTI) Vs. S&P 500: Which Is Better?
Lead Price Per Ounce Today & Historical Data

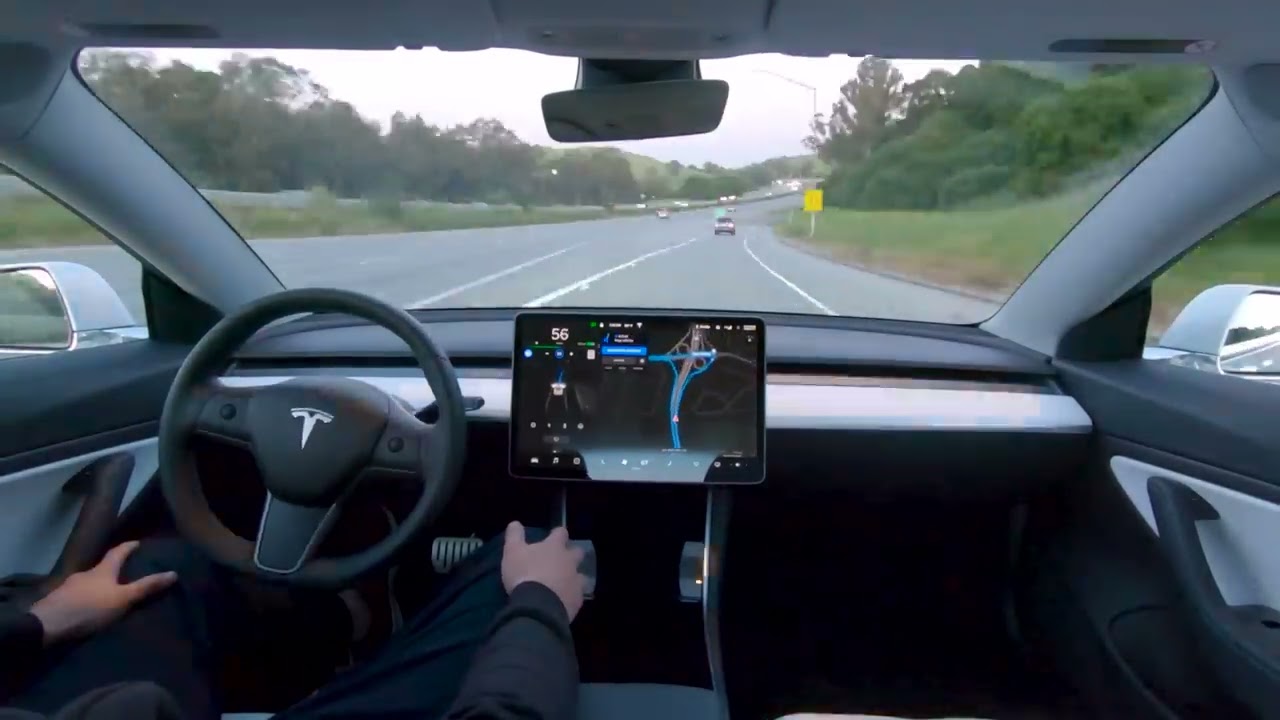
